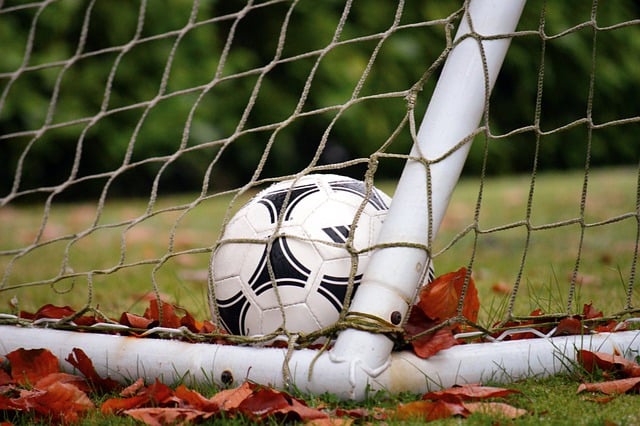
Expected goals (xG) statistics have significantly changed the way football is analyzed, providing deeper insights into team and player performance. There are several key ways in which xG has impacted football analysis.
Introduction to Expected Goals (xG) Statistics
Football analysis has changed dramatically in recent years thanks to advanced statistical models. One of the most important metrics used to assess team and player efficiency is xG (expected goals). This metric provides insight into the quality of chances a team creates and allows for a more objective assessment of match outcomes.
What is xG (Expected Goals)?
xG is an advanced statistical model that measures the probability of a shot resulting in a goal. Each shot is assigned a value between 0.01 and 1, where a higher value indicates a higher chance of scoring. The model takes into account various factors such as the angle of the shot, distance from goal, the position of opposing players, and the goalkeeper’s position.
The Difference Between xG and Traditional Statistics
Traditional football statistics, such as possession and shot count, do not provide a complete picture of a team’s efficiency. xG, on the other hand, allows for a deeper analysis because it measures not just the quantity of chances but also the quality of shots across the whole team. For example, a team may have a high number of shots, but if those shots are taken from unfavorable positions, their xG will be low.
Advanced xG Statistics
In addition to the basic xG metric, football analysts have developed additional indicators for even more precise match and player analysis:
- xG Scored: The number of goals a team should have scored based on the chances created.
- xG Conceded: The number of goals a team should have conceded based on the chances allowed to the opponent.
- xG Fairness: A metric comparing the actual result with the expected result to assess if the team deserved to win.
- xG Luckiness: Shows how much luck a team had in relation to expected goals.
- xGOT (Expected Goals on Target): Focuses only on shots on target and factors in shot strength, speed, and trajectory.
- xG by Halves: Breaks down expected goals by the first and second halves to analyze team performance throughout the match.
- xG Open Play: Calculates expected goals from open play (excluding set pieces).
- xG Set Play: Analyzes chances created from set pieces (e.g., free kicks, corners).
- xG Penalty: Assigns a value of 0.79 for each penalty, corresponding to the average penalty conversion rate.
- xPTS (Expected Points): Predicts how many points a team should have earned based on xG values.
- xG Predictability: Assesses how easy it is to predict a team’s results based on its xG performance.
How xG is Used in Match Analysis and Predictions
xG has changed the way football matches are analyzed, enabling more accurate assessments of team and individual performances. By analyzing xG values, it is possible to better understand which teams dominate in creating chances and which teams struggle to convert them.
Improving Player Performance Evaluation
Using xG, analysts can more precisely evaluate the quality of strikers and midfielders. A player who scores more goals than their xG suggests may be considered an efficient finisher, while a player with a high xG but few goals may have finishing issues.
Highlighting Team Strengths and Weaknesses
xG allows for an analysis of a team’s overall play, showing how the team creates and uses chances. A team with high xG but few goals may struggle with efficiency, while a team with low xG but many goals could be relying on luck or individual striker quality.
How Coaches and Analysts Use xG
Coaches and analysts use xG to analyze opponents, develop match strategies, and identify key players. Through xG, weaknesses in the opponent’s play can be spotted and exploited.
Optimizing Tactics and Strategy
By using xG analysis, coaches can adjust their team’s tactics. For instance, if xG shows a team creates many chances but fails to capitalize on them, the coach can focus on improving finishing or change the attacking formation.
Tracking Player and Team Progress
Long-term xG analysis allows for tracking player and team development. Players with a consistent increase in xG can be seen as improving in chance creation and overall play, while teams with consistently high xG can expect good results in the long run.
Criticism and Challenges in Using xG in Football
Although expected goals (xG) statistics have significantly improved football analysis, they are not without their criticisms and challenges. One of the main issues is the variability between different xG models, as various companies use their own algorithms and parameters to calculate expected goals. This means that data from different sources may differ, making consistent comparisons difficult.
Additionally, xG does not account for the quality of the shooter – two identical shots may have the same xG, but an elite striker is more likely to score than an average player. Furthermore, xG does not measure critical factors such as psychological pressure, defensive positioning at the moment of the shot, or weather conditions affecting the game.
Limitations and Drawbacks of xG Statistics
One of the main limitations of xG is that it is based on data from previous matches and does not take into account a player’s creativity or individual skills. Players with exceptional technical abilities often score from low-xG situations, meaning that the statistic does not fully reflect their quality.
Another drawback is the inability to accurately predict match outcomes. A team with a higher xG does not necessarily win, as matches are also decided by defensive abilities, tactical adjustments, and individual moments of inspiration. Moreover, xG does not measure defensive aspects of the game, such as a team’s ability to neutralize the opponent’s chances or pressing efficiency.
The Future of xG in Football Analysis
Despite its limitations, xG continues to evolve and improve. Modern analytical tools increasingly include advanced variations of xG, such as:
- xGOT (expected goals on target),
- xG Luckiness (goal-scoring luck),
- xG Predictability (xG model predictability).
Future models are expected to be even more accurate, thanks to the use of artificial intelligence and machine learning, which can analyze a broader range of game factors, including player positioning in real-time. In addition, xG could become a key tool in scouting and player evaluation, as it allows clubs to identify players who create chances from high-quality situations, even if they don’t score many goals.
Changing the Way Matches Are Analyzed with xG
xG is a revolutionary statistic that has changed the way football matches are analyzed. Thanks to xG, we can better understand team and player performances, predict future results, and clarify whether a result was deserved or a product of luck. While xG is not a perfect metric, its combination with other advanced statistical models provides invaluable value for the analysis of modern football.